Object Detection with Transformer#
Object detection เป็นการตรวจจับวัตถุต่างๆ (คน, สิ่งก่อสร่้าง, รถ) โดยโมเดลจะรับค่ามาเป็นรูปภาพและจะทำนายว่าวัตถุที่เราสนใจนั้นอยู่ตรงส่วนไหนของภาพและวัตถุนั้นจัดอยู่ใน class ใด object detection ถูกใช้ในงานหลากหลายรูปแบบเช่น autonomous driving ที่ใช้โมเดลในการตรวจจับบุคคล, เส้นถนน หรือ สัญญาณไฟ
Tutorial from : https://huggingface.co/docs/transformers/tasks/object_detection
# install related libraries
!pip install -q datasets transformers evaluate timm albumentations
!pip install transformers[torch]
━━━━━━━━━━━━━━━━━━━━━━━━━━━━━━━━━━━━━━━ 486.2/486.2 kB 9.7 MB/s eta 0:00:00
━━━━━━━━━━━━━━━━━━━━━━━━━━━━━━━━━━━━━━━━ 7.2/7.2 MB 70.9 MB/s eta 0:00:00
━━━━━━━━━━━━━━━━━━━━━━━━━━━━━━━━━━━━━━━━ 81.4/81.4 kB 6.2 MB/s eta 0:00:00
━━━━━━━━━━━━━━━━━━━━━━━━━━━━━━━━━━━━━━━━ 2.2/2.2 MB 40.7 MB/s eta 0:00:00
━━━━━━━━━━━━━━━━━━━━━━━━━━━━━━━━━━━━━━━ 110.5/110.5 kB 6.7 MB/s eta 0:00:00
━━━━━━━━━━━━━━━━━━━━━━━━━━━━━━━━━━━━━━ 212.5/212.5 kB 12.7 MB/s eta 0:00:00
━━━━━━━━━━━━━━━━━━━━━━━━━━━━━━━━━━━━━━ 134.3/134.3 kB 12.4 MB/s eta 0:00:00
━━━━━━━━━━━━━━━━━━━━━━━━━━━━━━━━━━━━━━ 236.8/236.8 kB 27.8 MB/s eta 0:00:00
━━━━━━━━━━━━━━━━━━━━━━━━━━━━━━━━━━━━━━━━ 7.8/7.8 MB 110.4 MB/s eta 0:00:00
━━━━━━━━━━━━━━━━━━━━━━━━━━━━━━━━━━━━━━━━ 1.3/1.3 MB 93.7 MB/s eta 0:00:00
?25hRequirement already satisfied: transformers[torch] in /usr/local/lib/python3.10/dist-packages (4.30.2)
Requirement already satisfied: filelock in /usr/local/lib/python3.10/dist-packages (from transformers[torch]) (3.12.2)
Requirement already satisfied: huggingface-hub<1.0,>=0.14.1 in /usr/local/lib/python3.10/dist-packages (from transformers[torch]) (0.15.1)
Requirement already satisfied: numpy>=1.17 in /usr/local/lib/python3.10/dist-packages (from transformers[torch]) (1.22.4)
Requirement already satisfied: packaging>=20.0 in /usr/local/lib/python3.10/dist-packages (from transformers[torch]) (23.1)
Requirement already satisfied: pyyaml>=5.1 in /usr/local/lib/python3.10/dist-packages (from transformers[torch]) (6.0)
Requirement already satisfied: regex!=2019.12.17 in /usr/local/lib/python3.10/dist-packages (from transformers[torch]) (2022.10.31)
Requirement already satisfied: requests in /usr/local/lib/python3.10/dist-packages (from transformers[torch]) (2.27.1)
Requirement already satisfied: tokenizers!=0.11.3,<0.14,>=0.11.1 in /usr/local/lib/python3.10/dist-packages (from transformers[torch]) (0.13.3)
Requirement already satisfied: safetensors>=0.3.1 in /usr/local/lib/python3.10/dist-packages (from transformers[torch]) (0.3.1)
Requirement already satisfied: tqdm>=4.27 in /usr/local/lib/python3.10/dist-packages (from transformers[torch]) (4.65.0)
Requirement already satisfied: torch!=1.12.0,>=1.9 in /usr/local/lib/python3.10/dist-packages (from transformers[torch]) (2.0.1+cu118)
Collecting accelerate>=0.20.2 (from transformers[torch])
Downloading accelerate-0.20.3-py3-none-any.whl (227 kB)
━━━━━━━━━━━━━━━━━━━━━━━━━━━━━━━━━━━━━━━ 227.6/227.6 kB 6.1 MB/s eta 0:00:00
?25hRequirement already satisfied: psutil in /usr/local/lib/python3.10/dist-packages (from accelerate>=0.20.2->transformers[torch]) (5.9.5)
Requirement already satisfied: fsspec in /usr/local/lib/python3.10/dist-packages (from huggingface-hub<1.0,>=0.14.1->transformers[torch]) (2023.6.0)
Requirement already satisfied: typing-extensions>=3.7.4.3 in /usr/local/lib/python3.10/dist-packages (from huggingface-hub<1.0,>=0.14.1->transformers[torch]) (4.6.3)
Requirement already satisfied: sympy in /usr/local/lib/python3.10/dist-packages (from torch!=1.12.0,>=1.9->transformers[torch]) (1.11.1)
Requirement already satisfied: networkx in /usr/local/lib/python3.10/dist-packages (from torch!=1.12.0,>=1.9->transformers[torch]) (3.1)
Requirement already satisfied: jinja2 in /usr/local/lib/python3.10/dist-packages (from torch!=1.12.0,>=1.9->transformers[torch]) (3.1.2)
Requirement already satisfied: triton==2.0.0 in /usr/local/lib/python3.10/dist-packages (from torch!=1.12.0,>=1.9->transformers[torch]) (2.0.0)
Requirement already satisfied: cmake in /usr/local/lib/python3.10/dist-packages (from triton==2.0.0->torch!=1.12.0,>=1.9->transformers[torch]) (3.25.2)
Requirement already satisfied: lit in /usr/local/lib/python3.10/dist-packages (from triton==2.0.0->torch!=1.12.0,>=1.9->transformers[torch]) (16.0.6)
Requirement already satisfied: urllib3<1.27,>=1.21.1 in /usr/local/lib/python3.10/dist-packages (from requests->transformers[torch]) (1.26.16)
Requirement already satisfied: certifi>=2017.4.17 in /usr/local/lib/python3.10/dist-packages (from requests->transformers[torch]) (2023.5.7)
Requirement already satisfied: charset-normalizer~=2.0.0 in /usr/local/lib/python3.10/dist-packages (from requests->transformers[torch]) (2.0.12)
Requirement already satisfied: idna<4,>=2.5 in /usr/local/lib/python3.10/dist-packages (from requests->transformers[torch]) (3.4)
Requirement already satisfied: MarkupSafe>=2.0 in /usr/local/lib/python3.10/dist-packages (from jinja2->torch!=1.12.0,>=1.9->transformers[torch]) (2.1.3)
Requirement already satisfied: mpmath>=0.19 in /usr/local/lib/python3.10/dist-packages (from sympy->torch!=1.12.0,>=1.9->transformers[torch]) (1.3.0)
Installing collected packages: accelerate
Successfully installed accelerate-0.20.3
Import libraries#
ที่ใช้ในการทำงาน เราจะใช้ transformers
และ datasets
มาช่วยในการทำงาน จาก huggingface hub
from transformers import AutoModelForObjectDetection # library สำหรับโหลด pre-trained model ที่เราต้องการ
from transformers import AutoImageProcessor # library สำหรับโมเดลที่เป็น image processor
from transformers import TrainingArguments # library สำหรับเก็บ arguments ที่เกี่ยวข้องกับการ train model
from transformers import Trainer # library สำหรับ train model
from datasets import load_dataset # library สำหรับโหลด dataset ที่เราต้องการ
import torch
import torchvision # library สำหรับการทำงานกับรูปภาพ
import evaluate # library สำหรับการคำนวณค่าความแม่นยำของโมเดล
from tqdm import tqdm # library สำหรับการแสดง progress bar
import albumentations # library สำหรับการทำ data augmentation
from PIL import Image, ImageDraw # library สำหรับการทำงานกับรูปภาพ
import requests # library สำหรับการดาวน์โหลดไฟล์จาก Google Drive (และอื่นๆ)
import numpy as np # library สำหรับการทำงานกับ array
import json # library สำหรับการทำงานกับไฟล์ json
import os # library สำหรับการทำงานกับไฟล์และโฟลเดอร์
Preparing Data#
Download cppe-5
dataset เพื่อใช้ในการ train model และ test model
cppe5 = load_dataset("cppe-5") # โหลด dataset ที่เราต้องการ
cppe5
Downloading and preparing dataset cppe-5/default to /root/.cache/huggingface/datasets/cppe-5/default/1.0.0/dd60c7c8210a67663b06108fb9e23c70acb98e2d3a4f3636f429509b19b74989...
Dataset cppe-5 downloaded and prepared to /root/.cache/huggingface/datasets/cppe-5/default/1.0.0/dd60c7c8210a67663b06108fb9e23c70acb98e2d3a4f3636f429509b19b74989. Subsequent calls will reuse this data.
DatasetDict({
train: Dataset({
features: ['image_id', 'image', 'width', 'height', 'objects'],
num_rows: 1000
})
test: Dataset({
features: ['image_id', 'image', 'width', 'height', 'objects'],
num_rows: 29
})
})
สร้าง id2label
และ label2id
ของ dataset ที่ใช้
categories = cppe5["train"].features["objects"].feature["category"].names # ดู category ทั้งหมดที่มีใน dataset
id2label = {index: x for index, x in enumerate(categories, start=0)} # สร้าง dictionary ที่ map จาก id ไปยัง label
label2id = {v: k for k, v in id2label.items()} # สร้าง dictionary ที่ map จาก label ไปยัง id
# remove images with no objects
remove_idx = [590, 821, 822, 875, 876, 878, 879] # ลบรูปภาพที่ไม่มี object ออกจาก dataset
keep = [i for i in range(len(cppe5["train"])) if i not in remove_idx] # สร้าง list ของ index ที่เราต้องการเก็บไว้
cppe5["train"] = cppe5["train"].select(keep) # ลบรูปภาพที่ไม่มี object ออกจาก dataset
Transform Data#
trasnfrom
data ก่อนที่จะเข้าไปใช้ใน model โดยใช้ albumentations
ในการทำ data augmentation
# สร้างขั้นตอนการแปลงรูปภาพเป็น feature vector โดยมีขั้นตอนดังนี้
# 1. ทำการ resize รูปภาพให้มีขนาด 480x480
# 2. ทำการกลับภาพแนวนอน (horizontal flip)
# 3. ทำการแปลง random brightness () และ contrast
# โดยที่ p คือความน่าจะเป็นที่จะทำการแปลง
transform = albumentations.Compose(
[
albumentations.Resize(480, 480), # resize to 480x480
albumentations.HorizontalFlip(p=1.0), # horizontal flip
albumentations.RandomBrightnessContrast(p=1.0), # random brightness and contrast
],
bbox_params=albumentations.BboxParams(format="coco", label_fields=["category"]), # กำหนด format ของ bounding box และ label
)
define function สำหรับการ train model
# formatted annotations
# ในการทำ annotation จะมี format ได้หลายรูปแบบโดยในที่นี้จะเป็น coco format
def formatted_anns(image_id, category, area, bbox):
annotations = []
for i in range(0, len(category)):
new_ann = {
"image_id": image_id,
"category_id": category[i],
"isCrowd": 0,
"area": area[i],
"bbox": list(bbox[i]),
}
annotations.append(new_ann)
return annotations
# transforming a batch
def transform_aug_ann(examples):
image_ids = examples["image_id"]
images, bboxes, area, categories = [], [], [], []
for image, objects in zip(examples["image"], examples["objects"]):
image = np.array(image.convert("RGB"))[:, :, ::-1]
out = transform(image=image, bboxes=objects["bbox"], category=objects["category"])
area.append(objects["area"])
images.append(out["image"])
bboxes.append(out["bboxes"])
categories.append(out["category"])
targets = [
{"image_id": id_, "annotations": formatted_anns(id_, cat_, ar_, box_)}
for id_, cat_, ar_, box_ in zip(image_ids, categories, area, bboxes)
]
return image_processor(images=images, annotations=targets, return_tensors="pt")
def collate_fn(batch):
pixel_values = [item["pixel_values"] for item in batch]
encoding = image_processor.pad_and_create_pixel_mask(pixel_values, return_tensors="pt") # ปรับขนาดของรูปภาพให้เหมาะสมกับโมเดล
labels = [item["labels"] for item in batch]
batch = {}
batch["pixel_values"] = encoding["pixel_values"]
batch["pixel_mask"] = encoding["pixel_mask"]
batch["labels"] = labels
return batch
# train from cppe5 model with augmentation
cppe5["train"] = cppe5["train"].with_transform(transform_aug_ann)
Create and Train a Model#
# เลือก pretrain_model และ image processor ที่จะใช้
checkpoint = "facebook/detr-resnet-50"
image_processor = AutoImageProcessor.from_pretrained(checkpoint)
Could not find image processor class in the image processor config or the model config. Loading based on pattern matching with the model's feature extractor configuration.
The `max_size` parameter is deprecated and will be removed in v4.26. Please specify in `size['longest_edge'] instead`.
# สร้าง model จาก pretrain_model ที่เลือก
model = AutoModelForObjectDetection.from_pretrained(
checkpoint,
id2label=id2label,
label2id=label2id,
ignore_mismatched_sizes=True,
)
Some weights of DetrForObjectDetection were not initialized from the model checkpoint at facebook/detr-resnet-50 and are newly initialized because the shapes did not match:
- class_labels_classifier.weight: found shape torch.Size([92, 256]) in the checkpoint and torch.Size([6, 256]) in the model instantiated
- class_labels_classifier.bias: found shape torch.Size([92]) in the checkpoint and torch.Size([6]) in the model instantiated
You should probably TRAIN this model on a down-stream task to be able to use it for predictions and inference.
กำหนด training_args
และ trainer
สำหรับการเทรนโมเดล
# [optional] disable wandb ที่ใช้ในการ log ข้อมูล
os.environ["WANDB_DISABLED"] = "true"
training_args = TrainingArguments(
output_dir="{directory_name}", # ชื่อโฟลเดอร์ที่เราจะเก็บ model ที่ train ได้
per_device_train_batch_size=2, # จำนวน batch size
num_train_epochs=10, # จำนวน epoch ที่เราต้องการให้โมเดล train
fp16=False, # ใช้ mixed precision หรือไม่
save_steps=200, # จำนวน step ที่เราต้องการให้โมเดล save
logging_steps=50,
learning_rate=1e-5, # ค่า learning rate
weight_decay=1e-4, # ค่า weight decay
save_total_limit=2, # จำนวน model ที่เราต้องการให้โมเดล save
remove_unused_columns=False,
push_to_hub=False,
)
Using the `WANDB_DISABLED` environment variable is deprecated and will be removed in v5. Use the --report_to flag to control the integrations used for logging result (for instance --report_to none).
# สร้าง trainer จาก model ที่เราสร้างไว้
trainer = Trainer(
model=model,
args=training_args,
data_collator=collate_fn,
train_dataset=cppe5["train"],
tokenizer=image_processor,
)
trainer.train() # train model
Step | Training Loss |
---|---|
50 | 2.076000 |
100 | 2.132500 |
150 | 1.933300 |
200 | 2.010700 |
250 | 1.989500 |
300 | 1.859800 |
350 | 2.015400 |
400 | 1.929600 |
450 | 1.872200 |
500 | 2.067200 |
550 | 1.849000 |
600 | 2.023300 |
650 | 1.971100 |
700 | 1.901000 |
750 | 1.849900 |
800 | 1.766900 |
850 | 1.831400 |
900 | 1.822200 |
950 | 1.837700 |
1000 | 1.681900 |
1050 | 1.716500 |
1100 | 1.715700 |
1150 | 1.739500 |
1200 | 1.606000 |
1250 | 1.788500 |
1300 | 1.709300 |
1350 | 1.846800 |
1400 | 1.579800 |
1450 | 1.547300 |
1500 | 1.808600 |
1550 | 1.700200 |
1600 | 1.689400 |
1650 | 1.620700 |
1700 | 1.542800 |
1750 | 1.704500 |
1800 | 1.568800 |
1850 | 1.573700 |
1900 | 1.532100 |
1950 | 1.490700 |
2000 | 1.598200 |
2050 | 1.516800 |
2100 | 1.485000 |
2150 | 1.471400 |
2200 | 1.589700 |
2250 | 1.477100 |
2300 | 1.572300 |
2350 | 1.630200 |
2400 | 1.605500 |
2450 | 1.449500 |
2500 | 1.529300 |
2550 | 1.547800 |
2600 | 1.447100 |
2650 | 1.405500 |
2700 | 1.488200 |
2750 | 1.436800 |
2800 | 1.550200 |
2850 | 1.359300 |
2900 | 1.551900 |
2950 | 1.542100 |
3000 | 1.443800 |
3050 | 1.367900 |
3100 | 1.531700 |
3150 | 1.395000 |
3200 | 1.450900 |
3250 | 1.421700 |
3300 | 1.604900 |
3350 | 1.285700 |
3400 | 1.496100 |
3450 | 1.324000 |
3500 | 1.416900 |
3550 | 1.453200 |
3600 | 1.484600 |
3650 | 1.364600 |
3700 | 1.384900 |
3750 | 1.393500 |
3800 | 1.307400 |
3850 | 1.436700 |
3900 | 1.291500 |
3950 | 1.382800 |
4000 | 1.318200 |
4050 | 1.499000 |
4100 | 1.368500 |
4150 | 1.450000 |
4200 | 1.245900 |
4250 | 1.414600 |
4300 | 1.418200 |
4350 | 1.344600 |
4400 | 1.340600 |
4450 | 1.309100 |
4500 | 1.371900 |
4550 | 1.426700 |
4600 | 1.318200 |
4650 | 1.329600 |
4700 | 1.403500 |
4750 | 1.306100 |
4800 | 1.294000 |
4850 | 1.477900 |
4900 | 1.272900 |
4950 | 1.290900 |
TrainOutput(global_step=4970, training_loss=1.5700076181883784, metrics={'train_runtime': 3326.617, 'train_samples_per_second': 2.985, 'train_steps_per_second': 1.494, 'total_flos': 4.7455894400256e+18, 'train_loss': 1.5700076181883784, 'epoch': 10.0})
Evaluate the Trained Model#
ทดสอบ model หรือ evaluation
เพื่อเช็คผลลัพธ์ของโมเดล
class CocoDetection(torchvision.datasets.CocoDetection):
def __init__(self, img_folder, feature_extractor, ann_file):
super().__init__(img_folder, ann_file)
self.feature_extractor = feature_extractor
def __getitem__(self, idx):
# read in PIL image and target in COCO format
img, target = super(CocoDetection, self).__getitem__(idx)
# preprocess image and target: converting target to DETR format,
# resizing + normalization of both image and target
image_id = self.ids[idx]
target = {"image_id": image_id, "annotations": target}
encoding = self.feature_extractor(images=img, annotations=target, return_tensors="pt")
pixel_values = encoding["pixel_values"].squeeze() # remove batch dimension
target = encoding["labels"][0] # remove batch dimension
return {"pixel_values": pixel_values, "labels": target}
# format annotations the same as for training, no need for data augmentation
def val_formatted_anns(image_id, objects):
annotations = []
for i in range(0, len(objects["id"])):
new_ann = {
"id": objects["id"][i],
"category_id": objects["category"][i],
"iscrowd": 0,
"image_id": image_id,
"area": objects["area"][i],
"bbox": objects["bbox"][i],
}
annotations.append(new_ann)
return annotations
# Save images and annotations into the files torchvision.datasets.CocoDetection expects
def save_cppe5_annotation_file_images(cppe5):
output_json = {}
path_output_cppe5 = f"{os.getcwd()}/cppe5/"
if not os.path.exists(path_output_cppe5):
os.makedirs(path_output_cppe5)
path_anno = os.path.join(path_output_cppe5, "cppe5_ann.json")
categories_json = [{"supercategory": "none", "id": id, "name": id2label[id]} for id in id2label]
output_json["images"] = []
output_json["annotations"] = []
for example in cppe5:
ann = val_formatted_anns(example["image_id"], example["objects"])
output_json["images"].append(
{
"id": example["image_id"],
"width": example["image"].width,
"height": example["image"].height,
"file_name": f"{example['image_id']}.png",
}
)
output_json["annotations"].extend(ann)
output_json["categories"] = categories_json
with open(path_anno, "w") as file:
json.dump(output_json, file, ensure_ascii=False, indent=4)
for im, img_id in zip(cppe5["image"], cppe5["image_id"]):
path_img = os.path.join(path_output_cppe5, f"{img_id}.png")
im.save(path_img)
return path_output_cppe5, path_anno
เลือก model
/checkpoint
ที่เราใช้ในการ evaluate
model_path = "{directory_name}/checkpoint-4800" # ใส่ directory ของ model ที่เราเทรนไว้
# เลือก processor จาก model ที่เรา train ไว้
im_processor = AutoImageProcessor.from_pretrained(f"{model_path}/preprocessor_config.json", local_files_only=True)
path_output_cppe5, path_anno = save_cppe5_annotation_file_images(cppe5["test"])
test_ds_coco_format = CocoDetection(path_output_cppe5, im_processor, path_anno)
# เลือก model ที่เรา train ไว้
model = AutoModelForObjectDetection.from_pretrained(f"{model_path}", local_files_only=True)
module = evaluate.load("ybelkada/cocoevaluate", coco=test_ds_coco_format.coco)
val_dataloader = torch.utils.data.DataLoader(
test_ds_coco_format, batch_size=2, shuffle=False, num_workers=0, collate_fn=collate_fn
)
# prediction และ evaluation
with torch.no_grad():
for idx, batch in enumerate(tqdm(val_dataloader)):
pixel_values = batch["pixel_values"]
pixel_mask = batch["pixel_mask"]
labels = [
{k: v for k, v in t.items()} for t in batch["labels"]
] # these are in DETR format, resized + normalized
# forward pass
outputs = model(pixel_values=pixel_values, pixel_mask=pixel_mask)
orig_target_sizes = torch.stack([target["orig_size"] for target in labels], dim=0)
results = im_processor.post_process(outputs, orig_target_sizes) # convert outputs of model to COCO api
module.add(prediction=results, reference=labels)
del batch
results = module.compute()
# แสดงผล evaluation
print(results)
loading annotations into memory...
Done (t=0.00s)
creating index...
index created!
100%|██████████| 15/15 [03:10<00:00, 12.67s/it]
Accumulating evaluation results...
DONE (t=0.09s).
IoU metric: bbox
Average Precision (AP) @[ IoU=0.50:0.95 | area= all | maxDets=100 ] = 0.194
Average Precision (AP) @[ IoU=0.50 | area= all | maxDets=100 ] = 0.351
Average Precision (AP) @[ IoU=0.75 | area= all | maxDets=100 ] = 0.164
Average Precision (AP) @[ IoU=0.50:0.95 | area= small | maxDets=100 ] = 0.036
Average Precision (AP) @[ IoU=0.50:0.95 | area=medium | maxDets=100 ] = 0.066
Average Precision (AP) @[ IoU=0.50:0.95 | area= large | maxDets=100 ] = 0.226
Average Recall (AR) @[ IoU=0.50:0.95 | area= all | maxDets= 1 ] = 0.176
Average Recall (AR) @[ IoU=0.50:0.95 | area= all | maxDets= 10 ] = 0.303
Average Recall (AR) @[ IoU=0.50:0.95 | area= all | maxDets=100 ] = 0.312
Average Recall (AR) @[ IoU=0.50:0.95 | area= small | maxDets=100 ] = 0.049
Average Recall (AR) @[ IoU=0.50:0.95 | area=medium | maxDets=100 ] = 0.143
Average Recall (AR) @[ IoU=0.50:0.95 | area= large | maxDets=100 ] = 0.326
{'iou_bbox': {'AP-IoU=0.50:0.95-area=all-maxDets=100': 0.1943336229049683, 'AP-IoU=0.50-area=all-maxDets=100': 0.3505048349605068, 'AP-IoU=0.75-area=all-maxDets=100': 0.163638553415943, 'AP-IoU=0.50:0.95-area=small-maxDets=100': 0.03597359735973597, 'AP-IoU=0.50:0.95-area=medium-maxDets=100': 0.06559100851470283, 'AP-IoU=0.50:0.95-area=large-maxDets=100': 0.22603634594743882, 'AR-IoU=0.50:0.95-area=all-maxDets=1': 0.17600840689365277, 'AR-IoU=0.50:0.95-area=all-maxDets=10': 0.3027007063439079, 'AR-IoU=0.50:0.95-area=all-maxDets=100': 0.31186183847225313, 'AR-IoU=0.50:0.95-area=small-maxDets=100': 0.048717948717948725, 'AR-IoU=0.50:0.95-area=medium-maxDets=100': 0.14334492753623188, 'AR-IoU=0.50:0.95-area=large-maxDets=100': 0.3257142857142857}}
Test the Model#
# เลือกรูปที่ต้องการ predict จาก url
url = "https://media.istockphoto.com/id/1221713778/photo/doctor-working-at-the-hospital-wearing-a-facemask-to-avoid-covid-19.jpg?s=612x612&w=0&k=20&c=EYJ7Kt9RtHLGqE6Fh8SY27D-mN3X--plXIvTgASABw0="
image = Image.open(requests.get(url, stream=True).raw)
display(image)
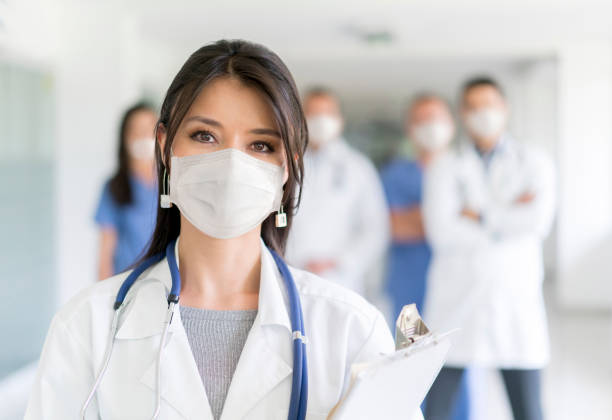
model_path = "{directory_name}/checkpoint-4800" # ใส่ directory ของ model ที่เราเทรนไว้
image_processor = AutoImageProcessor.from_pretrained(f"{model_path}/preprocessor_config.json", local_files_only=True) # processor
model = AutoModelForObjectDetection.from_pretrained(f"{model_path}", local_files_only=True) # model
# predict image
with torch.no_grad():
inputs = image_processor(images=image, return_tensors="pt")
outputs = model(**inputs)
target_sizes = torch.tensor([image.size[::-1]])
results = image_processor.post_process_object_detection(outputs, threshold=0.5, target_sizes=target_sizes)[0] # ใส่ threshold ที่เหมาะสม
thesold
เราสามารถเปลี่ยนค่าได้ตามความเหมาะสม เนื่องจากในบาง model ที่เราเทรนไว้ จะมีค่า confidence ของการ prediction
ที่ต่างกัน
# visualize results
for score, label, box in zip(results["scores"], results["labels"], results["boxes"]):
box = [round(i, 2) for i in box.tolist()]
print(
f"Detected {model.config.id2label[label.item()]} with confidence "
f"{round(score.item(), 3)} at location {box}"
)
Detected Mask with confidence 0.53 at location [302.52, 104.34, 347.88, 150.15]
Detected Mask with confidence 0.555 at location [406.68, 113.05, 453.15, 154.7]
Detected Mask with confidence 0.512 at location [459.08, 101.26, 505.28, 149.77]
Detected Mask with confidence 0.507 at location [454.49, 90.49, 507.94, 152.42]
Detected Mask with confidence 0.613 at location [168.46, 148.81, 288.17, 237.72]
Detected Coverall with confidence 0.892 at location [27.68, 37.03, 392.3, 418.04]
Detected Gloves with confidence 0.635 at location [390.18, 300.32, 433.65, 347.41]
Detected Coverall with confidence 0.777 at location [399.29, 68.52, 550.06, 416.53]
Visualize prediction โดยใช้ ImageDraw
draw = ImageDraw.Draw(image)
for score, label, box in zip(results["scores"], results["labels"], results["boxes"]):
box = [round(i, 2) for i in box.tolist()]
x, y, x2, y2 = tuple(box)
draw.rectangle((x, y, x2, y2), outline="red", width=1)
draw.text((x, y), model.config.id2label[label.item()], fill="white")
image
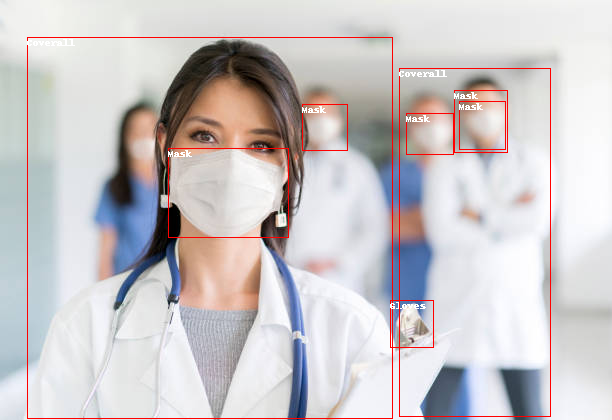
ผู้จัดเตรียม code ใน tutorial: ดร. ฐิติพัทธ อัชชะกุลวิสุทธิ์